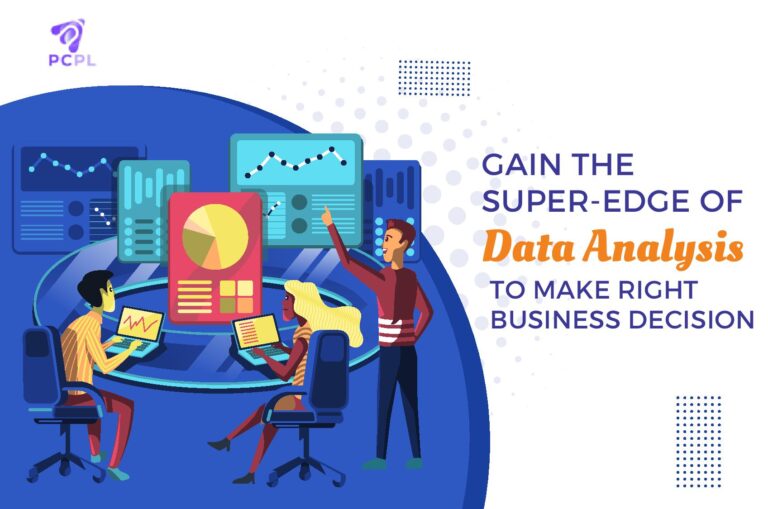
The Role of Data Analysis in Decision-Making: A Comprehensive Guide
In today’s data-driven world, organizations of all sizes are recognizing the immense value of data in making informed decisions. Data analysis has emerged as a critical process in extracting meaningful insights from raw data, enabling businesses to identify patterns, trends, and correlations that can guide strategic decision-making.
Understanding Data-Driven Decision Making
Data-driven decision-making refers to the process of making informed choices and taking actions based on objective analysis of relevant data. It involves collecting, analyzing, and interpreting data to extract meaningful insights that guide decision-making processes. By relying on data rather than intuition or assumptions, organizations can reduce biases, improve accuracy, and increase the chances of making optimal decisions.
Data-driven decision-making enables businesses to identify trends, patterns, and correlations, uncover hidden opportunities, mitigate risks, and drive strategic initiatives. It empowers decision-makers to rely on evidence-based insights, ultimately leading to better outcomes and a competitive edge in today’s data-driven world.
Understanding the Importance of Data Analysis
Types of Data Analysis Techniques
There are several techniques employed in data analysis, each serving a specific purpose. Some common types of data analysis techniques include:
Descriptive Analysis
Diagnostic Analysis
Diagnostic analysis aims to uncover the root causes of specific events or trends. By examining data and applying various statistical methods, it helps identify factors that contribute to observed outcomes, allowing organizations to address underlying issues.
Predictive Analysis
Predictive analysis makes use of statistical modeling techniques and historical data to predict future outcomes. By identifying patterns and relationships in data, organizations can make predictions about future trends, customer behavior, market dynamics, and more.
Prescriptive Analysis
Prescriptive analysis goes beyond predicting future outcomes. It suggests optimal courses of action based on the available data, taking into account constraints and objectives. It helps decision-makers explore different scenarios and make informed choices.
The Data Analysis Process
Data Collection
Gathering relevant data from various sources, ensuring data quality and accuracy.
Data Cleaning and Preparation
Cleaning and transforming the data to remove errors, inconsistencies, and missing values. This step also involves organizing and formatting the data for analysis.
Exploratory Data Analysis (EDA)
Statistical Analysis
Applying statistical methods to analyze data, test hypotheses, and draw meaningful conclusions. Techniques such as regression analysis, hypothesis testing, and correlation analysis are commonly used.
Data Visualization
Presenting the findings of the analysis through visual representations like charts, graphs, and dashboards to enhance understanding and facilitate decision-making.
Interpretation and Decision-Making
Interpreting the results of the analysis, extracting actionable insights, and using them to inform decision-making processes.
Tools and Technologies for Data Analysis
Data analysis relies on a variety of tools and technologies to handle and process vast amounts of data efficiently. Some popular tools include:
Spreadsheet Software
Tools like Microsoft Excel and Google Sheets offer basic data analysis capabilities, including sorting, filtering, and basic statistical functions.
Statistical Software
Business Intelligence (BI) Tools
BI tools like Tableau, Power BI, and QlikView enable businesses to create interactive visualizations, reports, and dashboards for data analysis and decision-making.
Machine Learning (ML) Platforms
ML platforms like TensorFlow and scikit-learn allow organizations to build predictive models and perform advanced data analysis tasks.
Challenges and Best Practices in Data Analysis
Data analysis comes with its own set of challenges, including data quality issues, data privacy concerns, and the need for skilled analysts. To ensure successful data analysis, organizations should adopt best practices such as:
Defining Clear Objectives
Data Governance
Data Visualization
Continuous Learning
Encourage a culture of continuous learning and upskilling among data analysts to keep up with evolving techniques and technologies.
Companies Who Put Analytics Into Practice
Companies across various industries harness the power of analytics to gain insights, improve operational efficiency, enhance customer experiences, and drive business growth.
Amazon
Netflix
Netflix is renowned for its data-driven approach to content recommendations and production. By analyzing user viewing patterns, ratings, and feedback, Netflix can tailor personalized recommendations to its subscribers. Additionally, they leverage data analysis to make strategic decisions in content creation and acquisition. Netflix analyzes viewer data to identify popular genres, cast members, and storylines, which helps them develop original content that aligns with audience preferences, increasing the likelihood of successful shows and attracting and retaining subscribers.
Uber