Benefits of Leveraging Machine Learning for Automation of Customer Sentiment
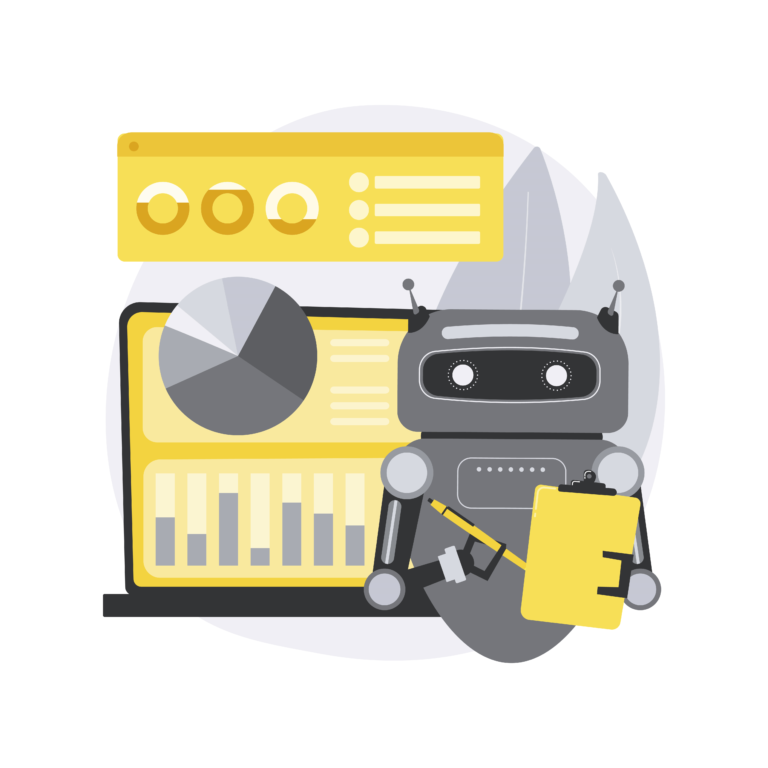
In today’s competitive business landscape, understanding the emotions and sentiments of your customers is essential to making informed decisions that will impact your business’s bottom line. With the rise of social media and online reviews, it has become more challenging for businesses to manually monitor and analyze customer feedback.
Fortunately, advancements in machine learning have made it possible to automate sentiment and emotion analysis, making it easier for businesses to gain insights into their customers’ feelings and opinions.
In this blog, we will explore how machine learning can be leveraged to automate customer sentiment and emotion analysis.
Sentiment Analysis
Sentiment analysis, also known as opinion mining, is the process of determining the emotional tone or attitude expressed in a piece of text. Sentiment analysis can be used to analyze social media posts, online reviews, customer feedback, and more. The sentiment of the text can be categorized as positive, negative, or neutral.
How to use Sentiment Analysis?
- Detect crises in their Infancy
- Constantly measure reputation trends
- Carry out Compepitive Analysis
Emotional Analysis
Emotion analysis is the process of identifying and extracting emotions expressed in a piece of text. Unlike sentiment analysis, which focuses on the overall sentiment of the text, emotion analysis delves deeper into the specific emotions expressed. Emotion analysis can be used to understand the emotional response of customers to a particular product or service.
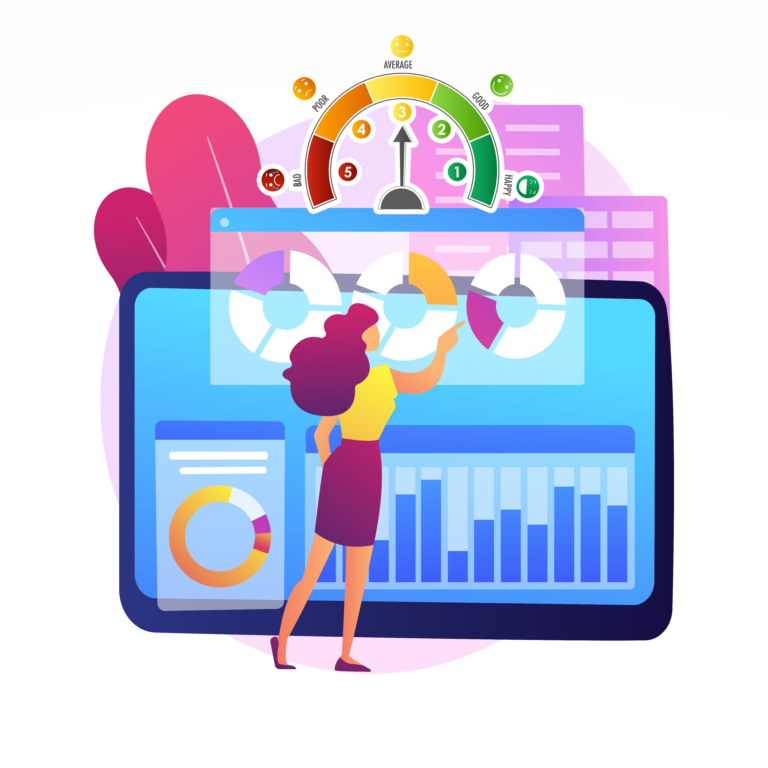
Automating Sentiment and Emotion Analysis with Machine Learning Ā
Machine learning algorithms can be trained to analyze text and identify patterns in the language that indicate sentiment or emotion. These algorithms use natural language processing (NLP) techniques to analyze the text and determine the sentiment or emotion expressed. NLP is a subfield of artificial intelligence that focuses on enabling machines to understand and process human language.
The machine learning algorithms are trained on large datasets of text that have been manually labeled with the appropriate sentiment or emotion. The algorithms use this labeled data to learn how to identify patterns in the language that indicates sentiment or emotion. Once the algorithms have been trained, they can be used to automatically analyze new text and determine the sentiment or emotion expressed.
Benefits of Automating Sentiment and Emotion Analysis
Automating sentiment and emotion analysis offers several benefits for businesses, including:
- Increased efficiency: Automating sentiment and emotion analysis saves time and resources compared to manual analysis.
- Improved accuracy: Machine learning algorithms can analyze large volumes of text accurately and quickly, reducing the risk of errors that can occur with manual analysis.
- Real-time analysis: With automated sentiment and emotion analysis, businesses can receive real-time insights into customer feedback, allowing them to respond quickly to any issues or concerns.
- Deeper insights: Emotion analysis goes beyond sentiment analysis, providing businesses with a more comprehensive understanding of their customers’ emotional responses.
Ā
Use Cases for Automated Sentiment and Emotion Analysis
Automated sentiment and emotion analysis can be applied to various use cases, including:
- Social media monitoring: Social media platforms are a rich source of customer feedback. Automated sentiment and emotion analysis can be used to monitor social media platforms and identify trends and patterns in customer feedback.
- Customer service: Automated sentiment and emotion analysis can be used to analyze customer feedback and identify areas for improvement in customer service.
- Product development: Emotion analysis can be used to understand how customers feel about a particular product or service, providing businesses with insights to inform product development.
- Brand management: Automated sentiment analysis can be used to monitor brand reputation and identify potential issues before they escalate.
Applications in Business
- Social media monitoring
- Customer support ticket analysis
- Brand monitoring
- Reputation management
- Listen to Voice of the Customer (VoC)
- Listen to voice of the employee
- Product analysis
- Market research
- Competitive research
Conclusion
Automated sentiment and emotion analysis using machine learning algorithms can provide businesses with valuable insights into their customers’ feelings and opinions. By automating sentiment and emotion analysis, businesses can increase efficiency, improve accuracy, and gain deeper insights into customer feedback. With the right tools and techniques, businesses can leverage machine learning to gain a competitive advantage and improve customer satisfaction.